Research
Prior to entering medicine, Dr. Chen had over a decade of experience and an advanced degree in biomedical engineering. Through medical school and residency, she continued to work on research projects that advance oncologic care through precision technology. Her work is published in several scientific and medical journals and she has presented at many national and international conferences.
Personalized prescriptions
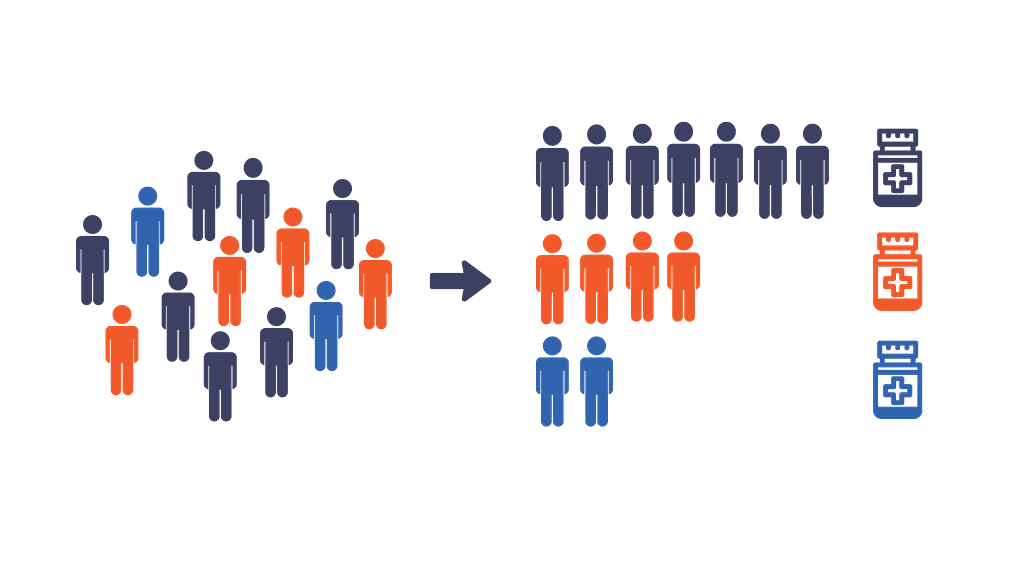
Personalized medicine tailors medical treatment to individual traits of each patient. We are all unique! A medication that works wonders for one person, may cause more harm than benefit in someone else. Dr. Chen’s work aimed to identify the relation between individual characteristics and efficacy of therapy using available scientific tools.
In radiation oncology, the prescribed dose is delivered to the tumor in “fractions”. In lung cancer, tumors located next to the esophagus and airways are called ultracentral tumors. Because of the proximity of sensitive structures to the tumor, the risks associated with radiation treatment is higher. These two patients received the same prescription dose and fractions. The treatment worked without any major side effects in one patient. In the other patient, the tumor grew back and they developed pneumonitis, an inflammation of the lungs. Dr. Chen’s research applied mathematical models to predict the prescription dose that would provide the best chance for tumor cure while minimizing the chance of treatment related side effects. This prescription dose may vary from patient to patient, based on their anatomy or medications they are taking at the time of radiation treatment. The team at Memorial Sloan Kettering Cancer Center leveraged this knowledge and predictive models to personalize the radiation treatment prescriptions for patients.

Radiomics

Radiomics is a portmanteau of ‘radio-’ from radiology and ‘-omics’ from words like genomics and proteomics. Medical images contain large amount of information, much more than what a radiologist can visually assess. A computer can extract these features such as texture and shape information. These features can be used to predict the course of disease or guide treatment decisions.
When cancer cells break away from the tumors in colon and rectal cancer, they most often form tumors or “metastasize” in the liver. Surgery is considered the treatment of choice for limited colorectal liver metastases. But it is not always the right treatment choice for every patient. In some patients, the risks of surgery don’t outweigh the benefit. These patients are offered localized treatment options, radiation therapy being one amongst these choices. Previous studies have shown that much higher doses of radiation are required to keep the cancer from returning in the liver for colorectal metastases compared to other types of cancers that metastasize to the liver. Using stereotactic body radiation therapy, or SBRT, very high dose of radiation can be delivered to the liver metastases. But even despite these high doses, in some patients tumors return after treatment. Dr. Chen worked with clinical faculty at Memorial Sloan Kettering Cancer Center and Simpson Lab at Queen’s University in Canada to use radiomics to understand the features that can be used to predict the response of this cancer type to radiation treatment.

Image guided therapy

Organ motion can present a problem in many fields of medicine – including surgery and radiation therapy. In surgery, patient imaging is done a day or two prior to the procedure and the scan is used in the image guidance system. Radiation treatments are based on a “simulation scan”, which is performed 1-2 weeks prior to treatment and the treatment is delivered over several days. Organs can move internally from time of simulation to treatment, and everyday between treatments. Internal organ motion can degrade the precision of therapy. Dr. Chen’s work has focused on different strategies to reduce these inaccuracies introduced by organ motion.
For brain tumor resection, the patient’s location in the operating room is mapped to the MRI image taken before the surgery by a mathematical process called “registration”. When the surgeon removes the bone flap, the fluid that bathes the brain, called cerebrospinal fluid or CSF, drains out and the gravitational force causes the brain tissue to sag. The neurosurgeons also give a medication called mannitol prior to surgery, which is meant to avoid brain swelling after the surgery. This medication causes overall shrinkage of the brain tissues. This “brain shift” can introduce errors upto several centimeters into the image guidance system. Dr. Chen worked with the team at Vanderbilt University on refining a biomechanical model that accounts for the error caused by brain shift and using this model to update the images used in the guidance system.

Radiation is sometimes used for treating pancreatic cancer that cannot be treated with surgery. The likelihood of preventing the cancer from returning depends on how high of radiation dose can be delivered to the tumor. But there are limits to how high this dose can be raised because of sensitive organs near the tumor, like small bowel. To further complicate matters, duodenum, the first part of the small bowel, can move between every treatment session. Dr. Chen’s work characterized the interfraction motion of the duodenum, meaning movement of this organ between each radiation treatment session, and found that motion can range up to several millimeters. She collaborated with the MR-guided radiation therapy team at the Department of Radiation Oncology in Washington University School of Medicine on utilizing MRI based image guidance to improve the accuracy of treatment delivery to mitigate the effect of internal organ motion.